Generative vs. General AI in Credit Management
Deciphering the Artificial Intelligence (AI) Debate
By Miriana Lowrie MICM
Artificial Intelligence (AI) has emerged as a cornerstone of innovation, reshaping the dynamics of multiple industries. Amara’s Law, put forward by the renowned technologist Roy Amara offers an insightful lens through which we can analyse the evolution and acceptance of emerging technologies.
This principle argues that society tends to overstate the short-term effects of a technology while underestimating its long-term implications. This is why we need to unravel AI’s Role in Credit’s Future – what it will and won’t be in the near future, and in the long term. Amidst the vast spectrum of AI technologies, the dawn of 2023 has seen one component pique everyone’s interest in an unprecedented manner – Generative AI. As we dissect the vast umbrella of AI, understanding AI, even partially, becomes crucial to grasp the evolving landscape and concepts. This leads us to delve deeper into what Generative AI is and why it’s garnering so much attention.
Unravelling the Mystery: What is Generative AI?
Generative AI refers to the subset of AI models that can generate new, previously unseen data that is similar in structure and style to the input data. A widely popularised model in this category is the Generative Adversarial Network (GAN), wherein two networks (Generator and Discriminator) work against each other, refining their outputs in the process.
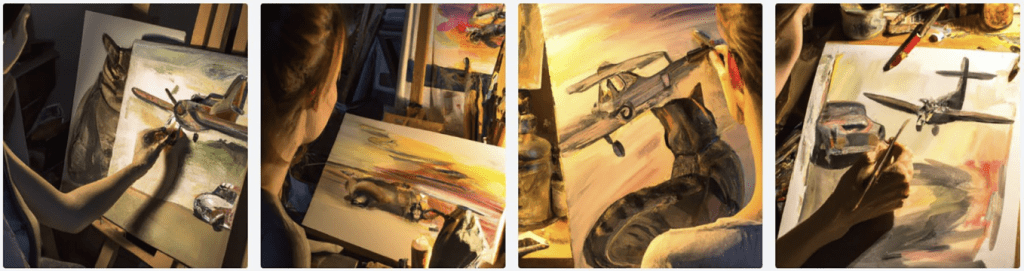
“Amidst the vast spectrum of AI technologies, the dawn of 2023 has seen one component pique everyone’s interest in an unprecedented manner – Generative AI.”
When you think about the realm of Generative AI, most will instinctively think about renowned models like ChatGPT, renowned for its prowess in text generation. However, the breadth of Generative AI’s application is expansive, stretching across domains from visual art, to music, literature composition, and even intricate design simulations.
In the creative arena, models like DeepDream from Google, turn boring images into psychedelic masterpieces, while MuseNet weaves enchanting musical compositions spanning a multitude of styles.
MidJourney enters the scene as a trailblazer, underscoring the limitless potential of generative techniques in creative processes. Building on the discussion of Generative AI and its wide-reaching applications, it’s essential to recognise that AI isn’t limited to just generative. Diverse subsets of AI offer capabilities ranging from creating novel content to understanding and interacting with the world around us.
AI Spectrum: There’s more than generative
While Generative AI centres around creation and simulation, another interesting area within the AI sphere focuses on interpretation and interaction. This area, known as Perception AI, aims to enable machines with capabilities that mirror human sensory perceptions. Let’s delve deeper into how machines can be taught to ‘experience’ their surroundings.
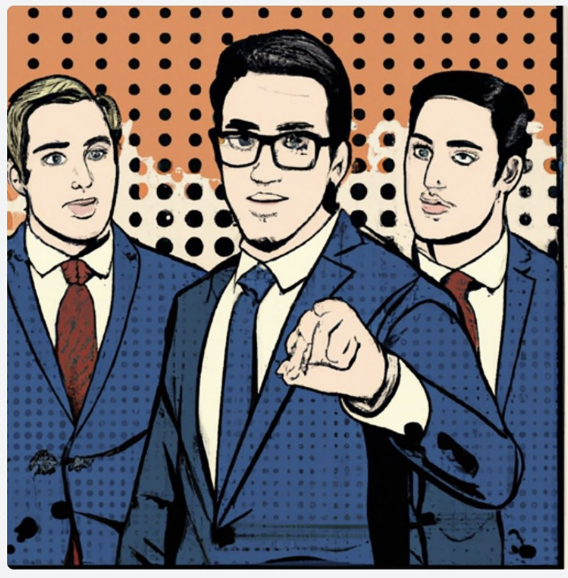
1. Perception AI: Understanding the World Around Us
Perception AI, at its core, is about machines comprehending the world in ways similar to human senses. Think of it as giving machines their own versions of sight, sound, and touch. This branch of AI allows computers to “perceive” or “sense” inputs from the real world, and then interpret and act on them.
USE CASE: AUTONOMOUS VEHICLES – Imagine a car that can see and interpret its surroundings. Using Computer Vision, our futuristic vehicle can detect and recognise obstacles, pedestrians, and signs. It’s like the car has eyes! With Speech Recognition, drivers communicate through voice, asking the car to play a song or set a route. Additionally, Sensor Fusion is the art of combining various sensor data (like radar or cameras) to understand the environment better. It’s the reason why self-driving cars won’t just stop because of a plastic bag floating across the road.
2. Learning and Prediction AI: Machines That Grow Smarter
This AI category is all about machines learning from data and predicting outcomes. Unlike traditional software that strictly follows coded instructions; these AI models adjust and improve over time based on the data they process.
USE CASE: ONLINE SHOPPING RECOMMENDATIONS – Have you ever wondered how online stores always seem to know what you want? Machine Learning (ML) observes your behaviour, like items you view or purchase, to suggest products. Generative AI can even craft personalised ads or product images based on your tastes. What are the intricate patterns that make these recommendations so accurate? That’s Deep Learning in action, diving deep into vast data to spot trends we humans might miss.
3. Reasoning and Decision Making AI: Logical Machines
This AI realm focuses on making logical decisions based on provided information. These systems can analyse vast amounts of data, consider various factors, and then make an informed decision or prediction based on logic.
USE CASE: FINANCIAL TRADING PLATFORMS – Expert Systems offer investment advice by following sets of predefined rules. Using Planning and Scheduling, these platforms can strategise trading tactics considering current market conditions. But it doesn’t stop there; cognitive architecture models the thinking processes of human traders, allowing AI to predict and decide in volatile markets. This just blows my mind.
4. Physical Interactions and Robotics: Machines in Motion
Beyond the digital realm, some AI interacts directly with our physical world. Robotics is where AI meets hardware, resulting in machines that can perform tasks, from the mundane to the highly specialised.
USE CASE: HOSPITAL-ASSISTIVE ROBOTS – Hospitals are increasingly relying on robots for various tasks. Using Robotics, these machines can carry supplies, sanitise rooms, or even assist in surgeries. Ensuring they work safely alongside humans, Human-Robot Interaction (HRI) allows these robots to understand our gestures and voice commands. And it’s not just about tasks; it’s also about movement. With Motion Planning, robots smoothly navigate busy hospital corridors without bumping into things
5. Language Processing AI: Bridging the Communication Gap
Language Processing AI is all about understanding and generating human language. It’s the tech behind those chatbots you talk to, the reason your voice assistant understands you, and the magic that helps you converse in a foreign language almost instantly.
USE CASE: VIRTUAL CUSTOMER SUPPORT – Ever chatted with a support bot? Natural Language Processing (NLP) is what helps it understand and respond to your queries. If you speak to it, Speech Processing turns your words into text, and vice versa. And if you ever interact with a bot in a foreign language, the seamless translation happening behind the scenes ensures everyone is on the same page.
Each of these AI categories provides a unique lens into how machines are mimicking, augmenting, or even surpassing human capabilities, reshaping industries and our everyday lives in the process.
“Whether it’s perceiving the world, understanding language, learning from data or making logical decisions AI continues to push the boundaries of what’s possible, not only in life, but also in Credit Management.”
Crunching the Numbers: AI’s Role in Credit Risk Management
Credit risk revolves around the inherent uncertainty of a party’s ability to fulfil its financial obligations. To steer through this uncertain terrain, credit risk management, fortified with AI tools, becomes paramount. It encompasses:
1. Exposure at Default:
This metric represents the amount at stake should a counterparty default. Traditional methods involve manual assessment of the total potential loss if a party experiences financial distress. However, with AI tools like regression algorithms, more accurate predictions can be made by analysing intricate patterns in vast datasets to determine the likely exposure.
2. Probability of Default:
At its core, this gauges the likelihood that the counterparty will default. Typically, evaluations use historical data, market conditions, and other indicators. But AI, especially deep learning models, can pore over massive volumes of data, discern subtle correlations, and improve the accuracy of default predictions. For instance, neural networks can be trained on extensive credit histories and transactional data to forecast potential default scenarios.
3. Loss Severity and Recovery Rate:
In the unfortunate event of a default, loss severity gives a measure of
the financial damage. The counterpart, recovery rate, sheds light on the recoverable portion of the owed amount. Integrating AI, algorithms can simulate various recovery scenarios, optimising strategies to maximise recovery. Machine learning models, when fed with historical recovery data, can forecast potential recovery outcomes with increased accuracy, guiding firms in decision-making. This, however, does take a mountain (as in Everest x100) of data to measure. Harnessing the vast potential of AI in credit risk management opens the door to a transformative shift in how the finance industry operates. It’s not just about enhancing the accuracy and reliability of credit evaluations but also adapting to the monumental shifts in data and consumer expectations.
Reaping the Rewards: Advantages of AI-driven Credit Management
AI techniques are rewriting the rulebook on credit management. Let’s delve into some of the most compelling advantages AI brings to the table, which collectively contribute to a future-ready credit management landscape. By integrating these, or at least some of these AI techniques, credit management becomes more proactive, precise, and personalised, ensuring a safer and more efficient financial landscape.
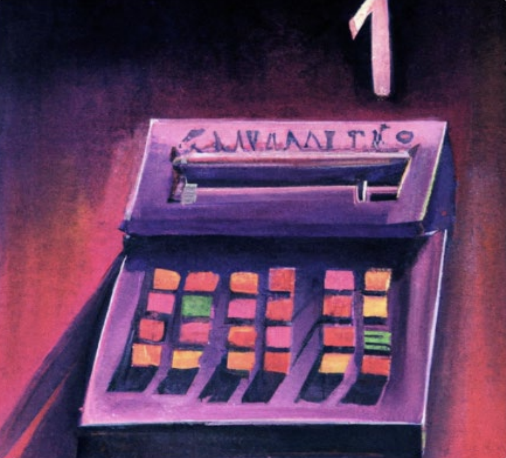
“Why has the intersection of AI and credit risk management become such a focal point in today’s discussions?”
1. Flexibility and Diverse Training Data:
AI’s adaptability means models can be trained across a wide spectrum of scenarios. Supervised learning, for example, utilises labelled data, such as past loan applications designated as ‘approved’ or ‘denied’, to make future predictions. Generative AI compliments this by generating diverse synthetic data, enhancing training sets for improved accuracy. To nail this down to industry and then region/state would be a really interesting scenario. For example – imagine your credit decision being determined by AI based on this data and not your own, or alongside your own credit policy? An interesting proposition.
2. Comprehensive Risk Assessment:
AI techniques provide comprehensive insights into potential risks. While Generative AI helps in understanding patterns beyond existing data, unsupervised learning methods, like clustering, segments customers based on transactional behaviour, unveiling deeper customer insights that can be crucial for risk assessments. CCCFA comes to mind when I think about this scenario.
3. Accurate Pattern Recognition and Prediction:
Neural networks and deep learning excel in discerning and predicting patterns. In credit management, this can translate to identifying potential defaulters or predicting market changes that can affect loan repayments. For example, a deep learning model might consider a myriad of variables, from a borrower’s transaction history to macroeconomic indicators, to predict creditworthiness. Think, preparing for a global pandemic, yes, we’ve all been here.
4. Fraud Detection and Prevention:
AI’s multi-faceted approach enhances fraud detection. Generative AI can simulate transactional patterns, while supervised learning can predict anomalies based on past fraudulent activities. Combined, they provide a robust mechanism to spot and prevent fraudulent transactions.
5. Tailored Credit Offerings:
AI’s ability to analyse and predict customer behaviour is revolutionary. Generative AI can simulate customer profiles, while unsupervised learning segments them based on behaviour. Together, they allow Lenders to design credit products tailored to individual needs, ensuring better customer satisfaction and reduced default rates.
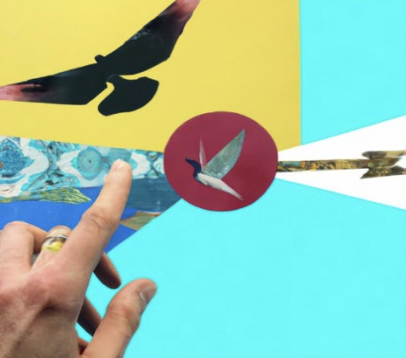
The Urgency of the AI Revolution in Credit Management
A Leap From Old Ways to a Data-Driven Era
While past credit decisions were predominantly influenced by historical behaviours and rudimentary models, the landscape is rapidly evolving. Every click, every online purchase, every social media post – our digital actions are producing a massive wave of behavioural data. This abundance is giving rise to cutting-edge credit decision-making tools, poised to fundamentally alter our lending and borrowing dynamics. The staggering volume of data generated in this era is reshaping traditional paradigms. AI and machine learning frameworks are here and will be transformative in how we approach credit risk management.
Evolving Consumer Expectations
Digital migration isn’t a one-way street. As we embed more of our routines online, we’re not only populating databases but also recalibrating our expectations. Modern consumers demand agile, bespoke financial services. Credit risk management, in response, must evolve. The focus must pivot from merely reflecting on past events to actively anticipating future challenges. With our mounting emphasis on expert data stewardship, the vision of AI-centric ‘risk robots’ helming this intricate domain is drawing ever closer. Is it the technologists and innovators that create this future?
The Millennial Factor
The tech-savvy millennial generation is ushering in a digital revolution. Their online behaviours are generating a goldmine of data, brimming with insights that could redefine risk assessment. And here’s an eye-catching stat: the global digital lending market is expected to skyrocket, growing at a CAGR of 53% and reaching a whopping $83.6 billion by 2025 (Zion market research, 2018). Why? Because digital platforms are letting lenders zoom in on potential borrowers with laser-like precision, offering tailored solutions at just the right moment. Imagine using AI to enable you to offer extended credit terms to those that qualify?
“What drives innovation? Technologists, customers or whomever has the biggest need?”
Trusting the Tech: How AI Compliments Human Insight in Credit Decisions
In essence, AI acts as an assistant, like a co-pilot, sometimes taking the lead in clear-cut scenarios and at other times signalling for a team huddle. It’s designed to optimise the credit decisioning process, be it through full automation for clear-cut cases or as a guiding tool that brings in human expertise when nuances come into play. The goal is a seamless, efficient, and informed approach to credit management, where AI and human judgement work in tandem. Let’s explore this in the context of fully automated and partially automated decisioning.
Fully Automated Decisioning
refers to the process wherein credit decisions are made instantly based on a predefined ruleset as determined by you. This offers the unbeatable advantage of speed, allowing for instantaneous decisions. Its consistency ensures that each decision, whether it’s for the hundredth or the thousandth application, is treated with the same set of rules, thereby drastically reducing human error. This approach shines particularly when scalability is in focus, as it can handle a vast number of applications without the need to scale up manpower. However, this streamlined method does come with its set of challenges. Its rigidity might sideline unique or outlier scenarios, and it remains wholly dependent on the accuracy and relevancy of the ruleset. If these rules become outdated or are flawed from the outset, the decisioning could be compromised.
Partially Automated Decisioning
on the other hand, strikes a balance between AI’s efficiency and human discernment. This method involves a blend of automated rules and human touch points. Credit applications are scanned and processed based on pre-established criteria. However, instead of instant approvals, specific scenarios or applications that trigger certain criteria are directed towards human approvers, informed by their delegation authorities. This ensures that complex or unique cases receive human oversight, offering a flexible approach to decisioning.
The benefit here is a marriage of speed and expertise.
Applications are processed rapidly, but there’s a layer of human oversight ensuring accuracy and discretion. This collaborative approach also allows various stakeholders to be part of the decision, fostering a more inclusive and informed decisioning process. But it’s worth noting that this method does lean on stakeholder availability, and as with any process involving human intervention, there’s a potential for inconsistencies or errors.
The Road Ahead: Navigating the Next Era of Credit Management
The marriage of AI and credit management is not just the future – it’s the present. With Generative AI shaping newer avenues and other AI methodologies enhancing traditional processes, the landscape of credit management is undergoing a radical transformation. By harnessing the power of these technologies, Lenders can aspire to achieve greater protection, efficiency, accuracy, and customer satisfaction. The challenge lies in responsible implementation, ensuring that the advances benefit both Lenders and Borrowers alike.
In the technology world, where AI holds centre stage, Amara’s Law serves as a timeless reminder. While the immediate promises of AI are indeed exhilarating, it’s the transformations and the untapped potential of this technology that will truly reshape our future.
Read more about AI and how it solves Credit Management headaches here.
References
- Zion Market Research. (2018). Global digitization in lending. Retrieved from https://www.zionmarketresearch.com/sample/digitization-in-lending-market
- Van Thiel, D., & van Raaij, W. F. (2019). Artificial intelligent credit risk prediction: An empirical study of analytical artificial intelligence tools for credit risk prediction in a digital era. Journal of Accounting and Finance, 19(8).
AI was used in the making of this article! The author extends gratitude to ChatGPT for assistance with content preparation and to ShutterstockAI for the creation of the accompanying imagery all of which used generative AI.
Read more articles related to this topic:
- Credit Assessment Has Been Same…
- What Enterprises Want From Trade-Credit Onboarding Platforms
- Business Case: Adopting a Trade-Credit Customer Onboarding Platform
- What do Netflix, Spotify, Salesforce, DocuSign and 1Centre all have in common?
- 5 factors to look for in your trade-credit software
- Three Big Conundrums for Today’s Credit Managers
- The importance of visibility in your sales pipeline
- 4 sales pipeline considerations for a new business